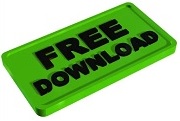

Because of their prevalence, organizational Linux-based virtual servers have become an attractive target for cyber-attacks, mainly launched by sophisticated malware designed at causing harm, sabotaging operations, obtaining data, or gaining financial profit. While different operating systems can run on top of VM instances, in public cloud environments the Linux operating system is used 90% of the time. Virtualization technologies, particularly virtual machines (VMs), are widely used and lie at the core of cloud computing. Since the beginning of the 21st century, the use of cloud computing has increased rapidly, and it currently plays a significant role among most organizations’ information technology (IT) infrastructure. Using a modified version of the registered ruleset of Snort, experimental results show that the maximum throughput of our IDS system can outperform that of Snort by a factor of 3 under many tested conditions. It examines the packets left by the first part to find the rules that match them. Its uses eBPF to perform fast patterns matching to pre-drop a very large portion of packets that have no chance to match any rule. In this work, we design and implement an IDS that has two parts working together. Recently, with the availability of Extended BPF (eBPF) in the Linux kernel, efficiently checking and filtering arriving packets directly in the kernel becomes feasible. Traditionally, an IDS, such as Snort, which is a widely used open source IDS, is implemented as a program running in the user space on a hardware server. It is an essential function for network security. This script takes in each log from the osquery and tries to match against various conditions to detect initial connections, lateral movement, and privilege escalation.Īn intrusion detection system (IDS) checks the content of headers and payload of packets to detect intrusions from the network.
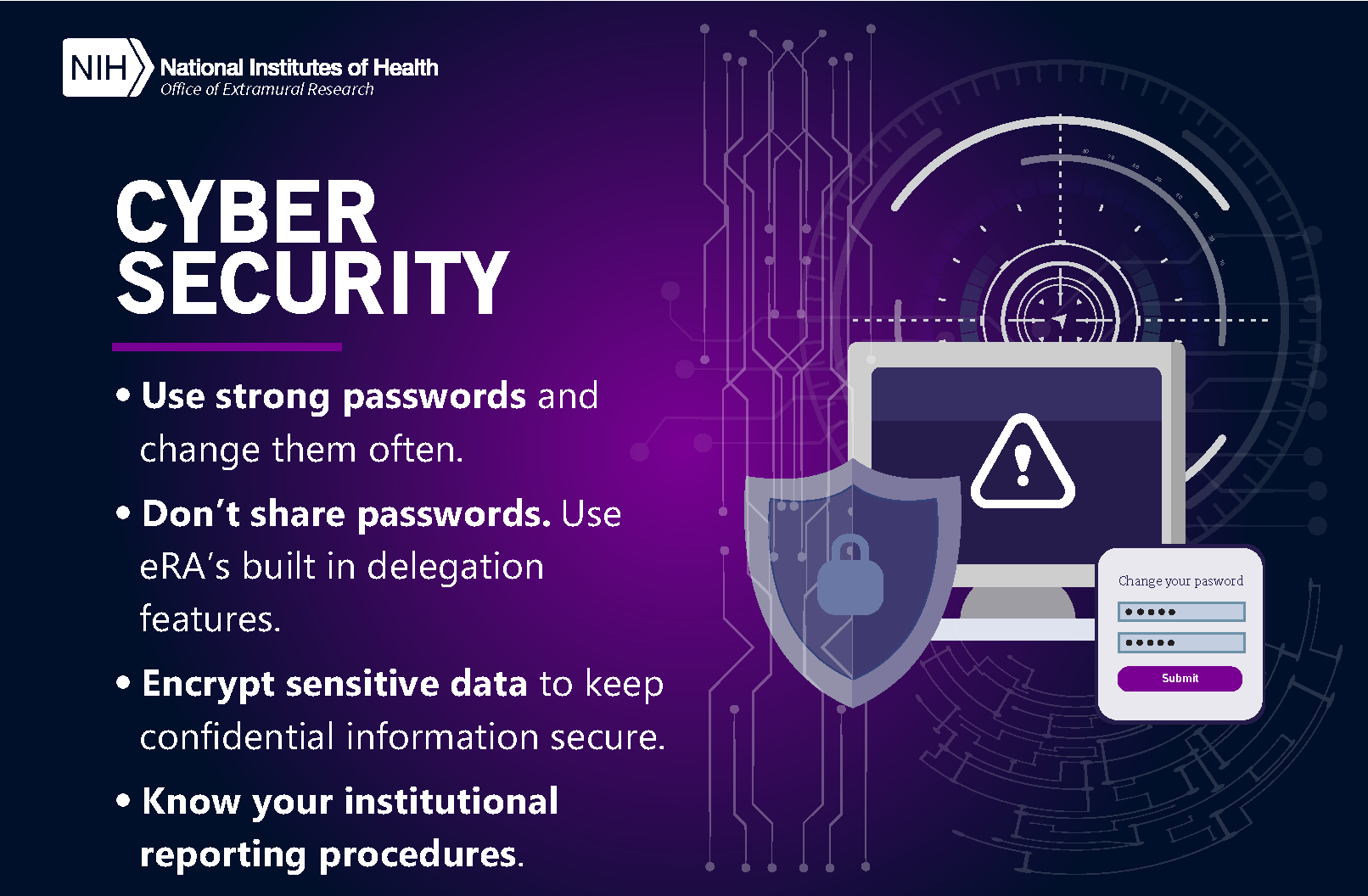
Using osquery, we were able to create a real-time heuristic-based detection script for Linux. This will allow us to provide detection in depth to machine learning models by detecting known bad that is sometimes missed by machine learning models. As majority of recent work focuses on machine learning to help detect attack, our focus of this paper is detection of attacks predominantly at the TTPs, Tools, and Network/Host Artifacts levels using heuristic-based detection. Detection can be done at multiple layers of David Bianco's Pyramid of Pain which consists of the following layers: TTPs, Tools, Network/Host Artifacts, Domain Names, IP Address, and Hash Values. With the increase in Unix-based operating system for web servers and IoT devices, it has become crucial to detect attacks that are performed on these critical devices.
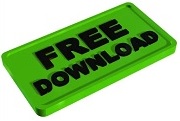